Cybersecurity
The Role of AI and Machine Learning in Managed Security
The complexity of cybersecurity concerns has increased in response to growing cyber threats, with attacks evolving at a rapid pace. Traditional security methods are struggling to keep up with this ever-changing landscape. Artificial Intelligence (AI) and Machine Learning (ML) have emerged as revolutionary powers, providing a proactive and adaptable defense against modern cyber threats. This transition is most visible in the emphasis on enhanced threat detection, which is an essential part of cybersecurity. Advanced threat detection, powered by AI and ML, goes beyond predefined rules, employing intelligent algorithms to study activities, discover abnormalities, and forecast potential threats before they occur. This proactive approach allows businesses to keep one step ahead of adversaries, reducing the impact of potential breaches.
In this blog, we’ll look at the definitions, distinctions, and overall impact of AI and machine learning on threat detection. We’ll look at how these technologies alter cybersecurity, from behavioral analysis for anomaly detection to AI’s predictive powers. The blog will showcase Machine Learning contributions such as pattern recognition and continuous learning, emphasizing their usefulness in uncovering previously undiscovered risks. Organizations can harden their defenses and foster a resilient security posture in the face of an ever-changing threat landscape by automating regular operations and incorporating AI and ML into managed security services.
To gain an in-depth understanding of the critical role that Artificial Intelligence (AI) and Machine Learning (ML) play in cybersecurity, it is critical to define and differentiate these words within the framework of managed security. Artificial Intelligence, in its broadest definition, refers to the use of machines to perform jobs that previously required human intelligence. Machine Learning, on the other hand, is a subset of AI that is primarily aimed towards systems learning from data and gradually improving their performance over time through iterative procedures.
The dynamic nature of modern cyber threats demands adaptive and intelligent security measures. Traditional approaches fall short in the face of rapidly evolving attack methodologies, emphasizing the need for advanced technologies that can keep pace with the ever-changing threat landscape. The combination of Artificial Intelligence (AI) and Machine Learning (ML) strengthens cybersecurity defenses by providing organizations with proactive and adaptive capabilities to manage the complex dynamics of today’s digital threat landscape.
Advancements in Explainable AI play a pivotal role in enhancing the transparency and interpretability of AI decisions, thereby fostering trust in technology, and enabling effective collaboration between humans and AI systems. By making AI more transparent and interpretable, organizations can gain deeper insights into AI-driven processes, facilitating informed decision-making and improving overall operational efficiency. Furthermore, exploring the concept of swarm intelligence in cybersecurity reveals the possibility of collective defense systems against coordinated cyber-attacks. Organizations can improve their security posture by leveraging collective intelligence to fight against sophisticated assaults that necessitate coordinated responses. This collaborative method taps into numerous entities’ cumulative knowledge and expertise, resulting in more effective threat identification and response.
Furthermore, the combination of AI and ML with upcoming technologies such as blockchain and quantum computing holds great promise for addressing difficult cybersecurity concerns. This synergy enables the creation of creative solutions that use each technology’s particular characteristics to improve cybersecurity defenses. Organizations can keep ahead of developing threats and effectively protect their digital assets from cyber-attacks by combining AI and machine learning with emerging technologies.
Definition: Understanding AI and Machine Learning in Managed Security
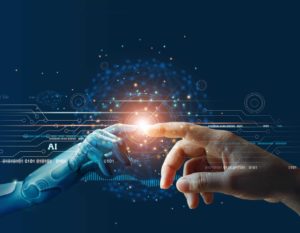
The Role of AI in Threat Detection
Artificial Intelligence (AI) revolutionizes cybersecurity through its multifaceted capabilities. Firstly, AI facilitates behavioral analysis for anomaly detection, efficiently recognizing patterns indicative of potential threats. AI systems use advanced algorithms to quickly recognize abnormalities from typical behavior, allowing for proactive threat mitigation. This predictive ability aids in the detection and prevention of security incidents. Furthermore, AI automation streamlines typical security activities, resulting in increased operational efficiency. This automation not only speeds up response times but also reduces the chance of human mistakes in repeated activities, allowing security teams to devote more time and resources to addressing difficult and strategic security concerns. AI serves as a foundation for strengthening cybersecurity defenses through these novel applications, providing organizations with the agility and foresight required to effectively navigate the growing digital threat landscape.Machine Learning’s Contribution to Threat Detection
Machine Learning (ML) is emerging as a key factor in cybersecurity, particularly due to its ability to recognize patterns in large datasets. This capability allows ML to detect and respond to threats based on previously learnt patterns, significantly improving the efficacy of threat detection and response methods. Furthermore, the concept of continuous learning is included in ML algorithms, ensuring flexibility to changing threat scenarios through continuing training. This dynamic learning approach enables systems to adapt in parallel with evolving threats, resulting in a strong defense mechanism. The ability of machine learning to identify previously unknown dangers, especially zero-day threats, represents a huge breakthrough. This capability directly addresses the issue given by quickly evolving attack strategies, hence increasing organizations’ resilience to emerging threats. ML’s pattern recognition and continuous learning capabilities are a strong asset in the cybersecurity armory, allowing enterprises to proactively protect against changing threats with better precision and efficiency.Challenges and Limitations
The challenge of bias in AI algorithms poses significant implications for security measures within cybersecurity contexts. Bias in AI refers to systemic flaws or inaccuracies in machine learning algorithms that lead to unfair or discriminatory results. These biases can come from a variety of sources, such as biased training data, algorithmic design decisions, or human prejudices entrenched in the development cycle. Bias can take many forms, including gender, racial, and cultural prejudices, and can lead to skewed results in danger detection and response. Strategies for reducing bias in threat detection are critical to maintaining fairness and efficacy in security operations. By tackling bias, organizations can improve the accuracy and reliability of AI-powered security solutions, thereby boosting their cybersecurity posture. Furthermore, the ability of threat actors to exploit and manipulate ML algorithms emphasizes the need for strong countermeasures to improve the resilience of ML-driven security systems. Threat actors may try to manipulate algorithms to avoid detection or launch targeted attacks, emphasizing the significance of proactive defenses. Continuous monitoring and algorithm updates are critical techniques for staying ahead of any manipulations and ensuring the integrity of security systems.Integrating AI and ML into Managed Security Services
A collaborative approach between AI/ML systems and human security analysts is essential. Striking a balance between automation and human expertise ensures comprehensive threat detection and response capabilities. Organizations must carefully consider compatibility with existing security infrastructure when choosing AI and ML solutions. The selection process should prioritize technologies that seamlessly integrate into the existing security ecosystem. The integration of AI and ML into managed security services necessitates training security teams on these advanced technologies. Building a workforce equipped to leverage AI and ML is essential for maximizing the potential of these technologies.Future Trends and Innovations
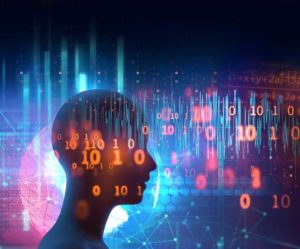